We spend 20% of our time searching for answers at work; what’s the solution?
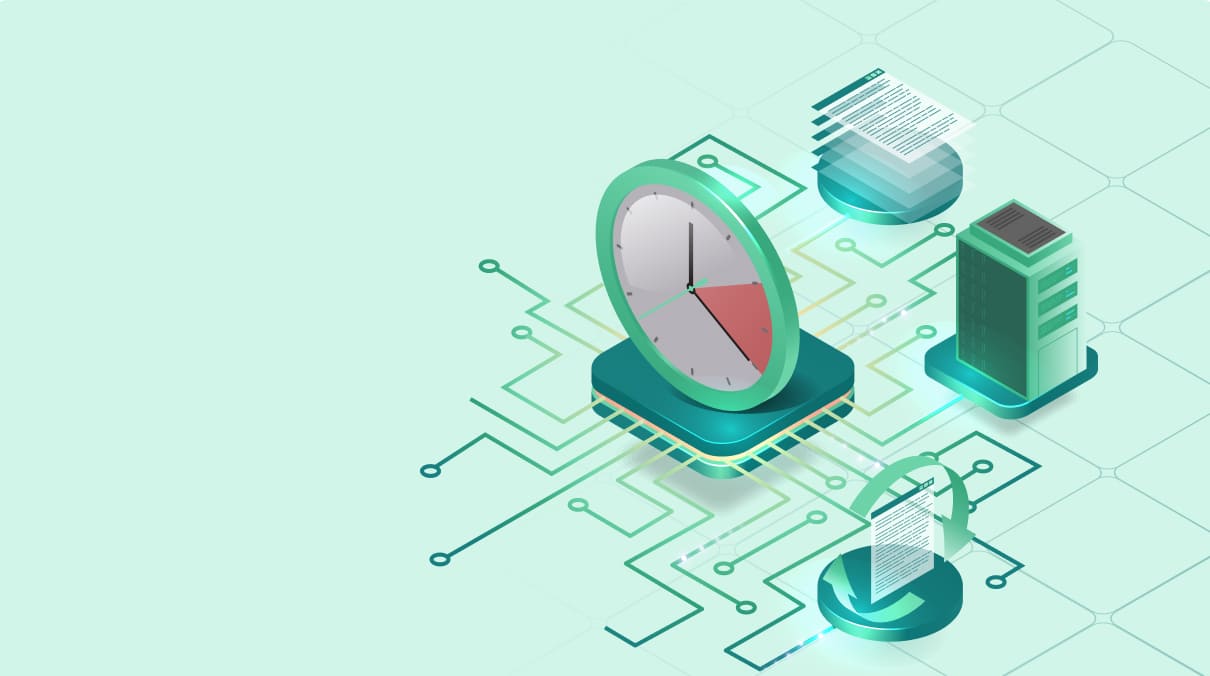
We’ve all been there. You’re gearing up for a major presentation, but you’re missing a crucial piece of information mentioned weeks ago.
You dive into your emails, chats, and content systems, desperately searching for it. Yet, despite your efforts, it remains elusive. You reach out to colleagues for help, but they come up empty-handed too.
This is the problem faced by knowledge workers (e.g. salespeople, marketers, engineers, etc.) all around the world.
According to a well-known McKinsey study, we spend an average of 1.8 hours per workday (about 20%) hunting down information. That adds up to a whopping 9.3 hours per week devoted solely to searching.
Change can be challenging, especially when everyone is constantly busy. However, if a significant portion of our busy time — say, one day a week — is spent searching for what we need to do our jobs, perhaps it’s time to address this issue.
We need to start caring about the 20%
If the statistic from McKinsey is true, what does it mean for companies?
For a business owner with a workforce of 100 knowledge workers, it’s like having only 80 of them show up for work. When you consider that 20 percent of the wage bill essentially covers unproductive work, the situation becomes concerning.
Caring about something is one thing, but it takes courage to speak up or take action.
Does this issue cause enough stress and frustration for us to discuss it with our boss? Or will we simply dismiss it as “just part of life”?
Using AI to unify scattered data
Thankfully, we live in an era where cutting-edge AI/ML tech understands language and semantic search to help workers quickly find work answers.
Many platforms are experimenting with this new technology. Notion, for example, recently launched a Q&A feature that acts as a search engine for content stored in the app.
Salesforce has its own AI-powered search engine, too. But while employees might find these new features useful, they still have to navigate between different interfaces to gain the insights they need to do their work
Trustworthiness and accuracy issues
One of the major concerns with current generative AI solutions, such as Copilot, is their propensity for inaccuracy.
For instance, when tasked with summarizing GAP’s financial data, Copilot completely misinterpreted key data points, leading to potentially costly business decisions. This issue of trustworthiness is critical because inaccurate data can lead to misguided strategies and operational inefficiencies.
Transparency and traceability
Another significant challenge is the opacity of AI decision-making processes.
Understanding how generative AI models produce answers is often impossible, which complicates their integration into environments where decision-making needs to be justified with concrete reasoning.
Additionally, most generative AI tools do not cite their sources, making it difficult for users to verify the information and trace it back to its origins.
Limitations in handling numerical data
Generative AI technologies also struggle with tasks involving numerical data and computations, commonly referred to as “hallucinating” incorrect figures.
This limitation is particularly problematic in fields like finance or any quantitative analysis where precision is crucial.
Data protection and authorization
The use of proprietary data to train public AI models raises significant concerns regarding data protection.
There is a risk of sensitive information being inadvertently exposed through AI training processes.
Furthermore, setting permissions within AI systems remains a challenge; for instance, ensuring that HR teams have access only to relevant data, without inadvertently exposing them to sensitive financial information, is not yet feasible with most AI platforms.
Customization and integration
Current generative AI solutions often lack sufficient customization options. Businesses cannot tailor these AI tools to reflect their unique brand identity or adjust the AI’s tone of voice to match their corporate communication style.
This lack of personalization can make AI feel like a foreign tool rather than an integrated part of the business.
Moreover, these AI systems frequently do not integrate well with existing enterprise systems like ERP, CRM, or HCM, which limits their practical utility in enhancing operational efficiencies across various business functions.
Recognizing contradictions and working with imperfect data
Finally, the current generation of AI lacks the sophistication to analyze contradictions or discrepancies across multiple data sources.
The ability to handle and rationalize imperfect data would significantly enhance decision-making processes, allowing companies to operate more dynamically and responsively.
Introducing Seraf: secure, accurate Gen AI solution
At Seraf, we’re building an AI chatbot that unifies all of your company data in a single platform. Employees can ask Seraf any questions they face in their day to day work and get instant answers back. This is because Seraf knows where that piece of information lives, and understands its context.
Seraf was built from the ground up with data governance in mind. Only the right people can see the right information and your data is never used to train public models.
Plus, the answers Seraf gives you are 100% hallucination-free, as Seraf uses advanced SQL techniques to generate insights and answers from your data.
Seraf’s interface is designed for ease of use. Employees can interact with Seraf as they would with a colleague, asking questions and receiving information that is not only accurate but immediately usable. This reduces the friction typically associated with adopting new technologies and accelerates the path to productivity.
Final thoughts
In conclusion, we all know how frustrating it can be to waste time hunting for information at work—it’s a big productivity killer.
Thankfully, with the latest AI and machine learning tools, we can cut down on this inefficiency significantly.
These technologies are not only making it easier and faster to find what we need but also ensuring that the data is accurate and well-integrated with our existing systems.
As these tools keep getting better, it’s crucial for companies to hop on board. This isn’t just about saving time; it’s about working smarter and making our workplaces more dynamic. It’s clear—embracing AI is the way forward.
Get valuable insights about AI and business automation
Rely on Seraf, AI that consolidates all your systems
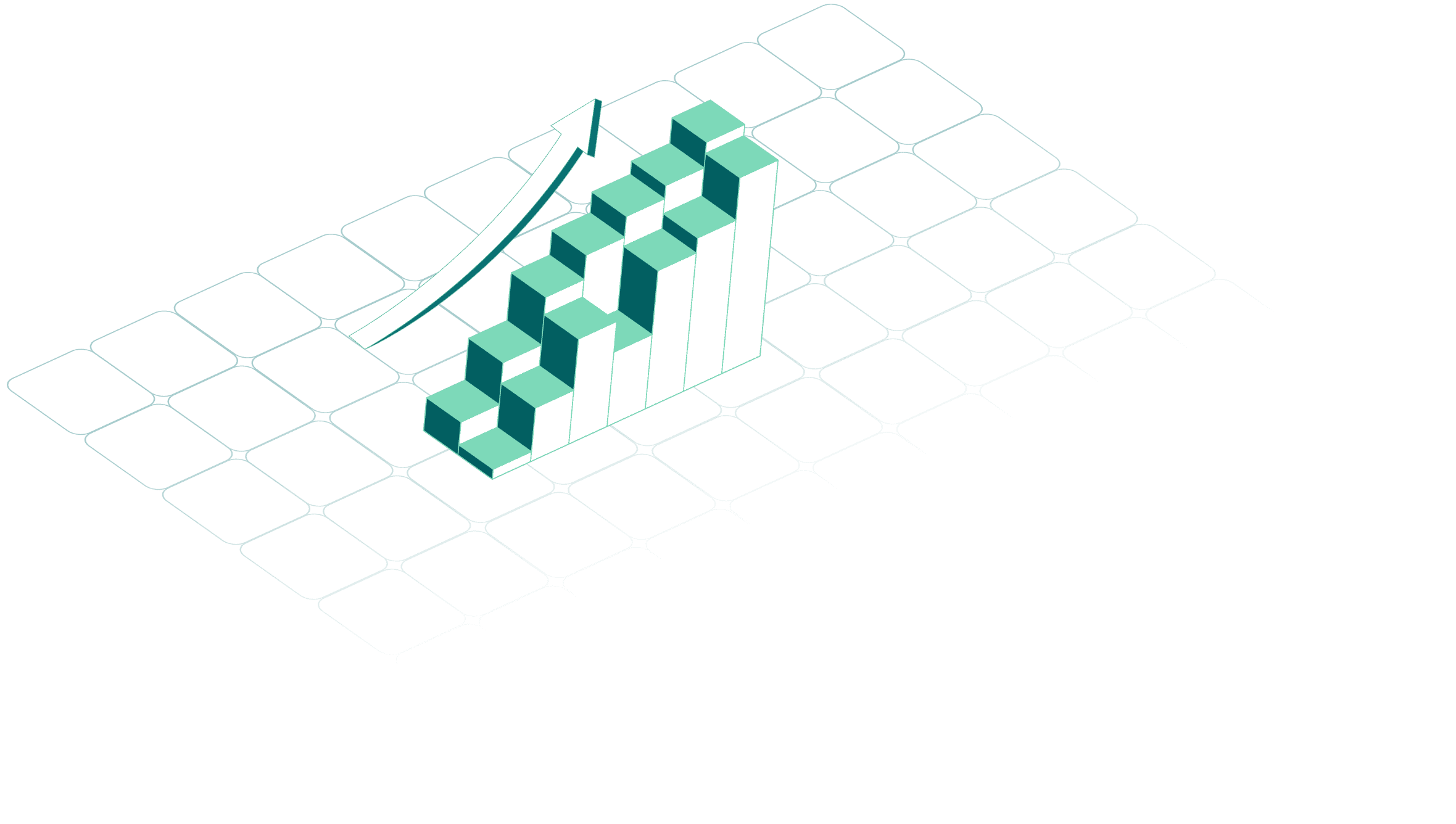